Multi-technology
Analyze most common Transcriptomics, Metabolomics, Proteomics data formats and integrate the results into one research project
Technological advancements in biomedical sample analysis have revolutionized our ability to decode the complexities of biological systems.
High-throughput sequencing, mass spectrometry, and imaging techniques now provide unprecedented levels of molecular detail. However, these advancements have also led to a proliferation of multiple data formats across omics disciplines, presenting significant challenges in data integration and interpretation. The power of cloud computing, no-code data analysis and easy meta-data standardization are pivotal in overcoming these challenges. By leveraging the UniApp, you can harmonize multi-omics data, enabling cross-platform analysis and holistic understanding to reveal complex biological interactions at unprecedented scales.
Key highlights
High-throughput multi-omics data analysis
Overcome most data integration challenges with meta-analysis
Wide array of supported data formats
High-throughput analysis of transcriptomics, metabolomics, proteomics data
Multi-omics refers to the collective technologies used to explore the roles, relationships, and actions of the various types of molecules that make up the cells of an organism. The UniApp platform provides extensive analysis tools for high-throughput transcriptomics, proteomics and metabolomics data. By combining these data types, you can achieve a more holistic view of the biological processes and mechanisms at play. The UniApp platform is optimized to fully leverage a growing array of data formats. Find out the latest details here.
Overcome most data integration challenges with meta-analysis
One of the questions encountered in multi-omics or large data studies pertains to data integration. While data integration is preferable in specific cases, we strongly believe in meta-analyses. A meta-analysis approach aggregates and analyzes summary statistics or effect sizes derived from individual studies. The key benefits of meta-analysis over data integration are summarized as follows:
- Aggregated Evidence: Meta-analysis synthesizes results from multiple studies, increasing statistical power to derive robust conclusions about treatment effects, risk factors, or biomarkers.
- Reduced Bias: By pooling data from multiple studies, meta-analysis can mitigate biases inherent in individual studies, such as publication bias and selective reporting of outcomes.
- Generalizability: Meta-analysis evaluates consistency and variability across studies, providing a broader perspective on the generalizability of research findings across different populations, settings, or methodologies.
- Efficiency: Conducting a meta-analysis can be more time-efficient and cost-effective compared to collecting and integrating raw data from multiple sources.
To efficiently conduct meta-analysis, two factors are critical: the ability to analyze individual datasets and integrate these results quickly, and specific sets of analysis and visualization options to derive meaningful insights from your meta-analysis. Learn more about UniApp’s specific features that support large meta-analysis studies in our client success stories or use case section.
Growing wide array of supported data formats (last update: June ’24)
Transcriptomics
- Microarray analysis: measuring gene expression levels using microarray chips
- Bulk-RNA sequencing (RNA-seq): quantifying gene expression by sequencing RNA transcripts
- Single-cell RNA sequencing (scRNA-seq): analyzing gene expression at the single-cell level to understand cellular heterogeneity
- Spatial Single-cell RNA sequencing (sscRNA-seq): combining single-cell RNA sequencing with spatial information to map gene expression in the context of tissue architecture
- CITE-seq: integrating Cellular Indexing of Transcriptomes and Epitopes by sequencing (CITE-seq) to simultaneously profile RNA and surface proteins at the single-cell level, allowing for a more comprehensive understanding of cellular states and functions.
Proteomics
- Shotgun proteomics: identify proteins in a complex mixture by digesting them into peptides and analyzing by Mass Spectrometry
- SILAC proteomics: incorporate Stable Isotope Labeling by Amino acids in Cell culture (SILAC) for quantitative proteomics, allowing for the comparison of protein abundances between samples
- MALDI-TOF: using Matrix-Assisted Laser Desorption/Ionization-Time of Flight (MALDI-TOF) mass spectrometry for the rapid identification and characterization of proteins, peptides, and other biomolecules based on their mass-to-charge ratio
Metabolomics
- Nuclear Magnetic Resonance (NMR) Spectroscopy: identifying and quantifying metabolites in biological samples
- Gas Chromatography-Mass Spectrometry (GC-MS): analyzing volatile and semi-volatile compounds
- Liquid Chromatography-Mass Spectrometry (LC-MS): identifying and quantifying metabolites in liquid samples
- Capillary Electrophoresis-Mass Spectrometry (CE-MS): analyzing charged metabolites and small molecules
Related modules
Cloud compute power
Leverage the power of cloud computing to drastically reduce analysis runtimes without specific hardware and tap into the potential of performing large data studies
Data visualizations
Explore your data with interactive and easy customizable data output visualizations without any coding or scripting

Project management tools
Build your research paper storyline with interactive figure panels readily to present while keeping track of data files, versions and tasks
Data organization
Gain control over your valuable data files in a single secured environment, allowing you to easily share data and collaborate
Proud to accelerate biomedical research at our clients
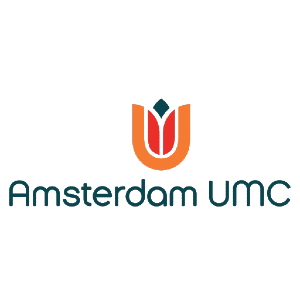
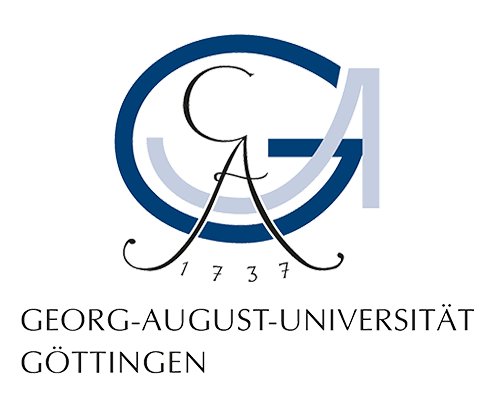
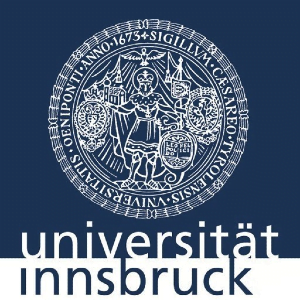
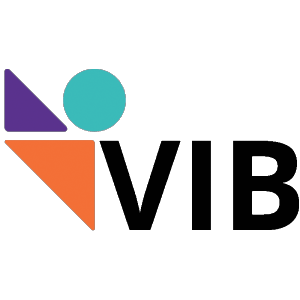
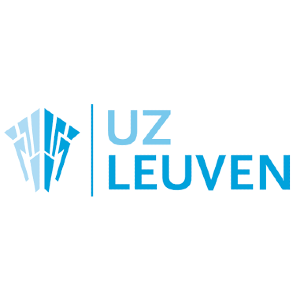
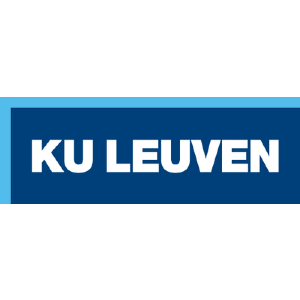
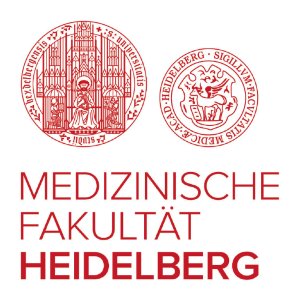
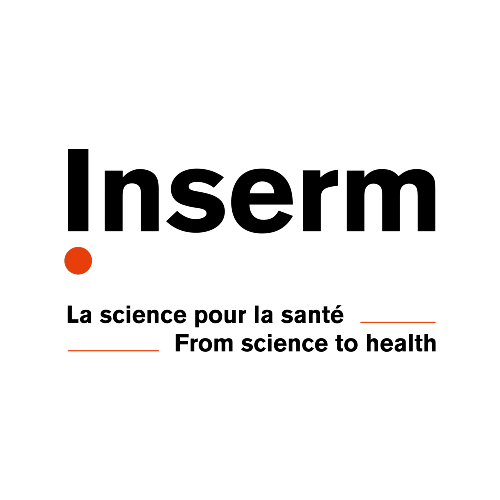
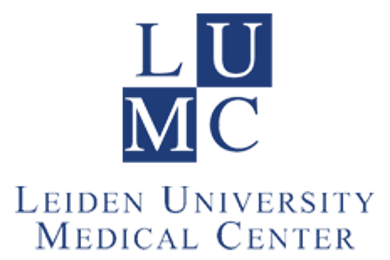